SCIENTIFIC ACHIEVEMENT
Researchers at the Advanced Light Source (ALS) showed that machine learning can predict noisy fluctuations in the size of beams generated by synchrotron light sources and correct them before they occur.
SIGNIFICANCE AND IMPACT
The work solves a decades-old problem and will allow researchers to fully exploit the smaller beams made possible by recent advances in light source technology.
A new approach to an old problem
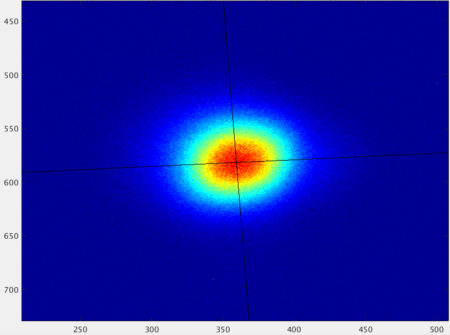
Many synchrotron facilities deliver light to dozens of beamlines simultaneously. One side effect of this is that the motions of certain insertion devices (IDs)—undulators and wigglers with variable magnetic fields—cause the size of the electron beam to fluctuate, affecting the performance of other beamlines. Typically, corrections for these fluctuations are arrived at through the use of static physics models of the ID magnetic fields and time-consuming calibration measurements. However, with the advent of diffraction-limited storage rings with dramatically smaller beam sizes, much tighter control over beam stability is needed.
Here, for the first time, researchers have demonstrated that a neural network (a computerized pattern-recognition algorithm) can “learn” to predict the beam size during regular operation, given sufficient data about how ID configurations affect the beam. The predictions can then be used to neutralize beam perturbations before they occur (“feed-forward” vs feed-back correction), achieving an order-of-magnitude improvement in stability that fulfills the requirements for future light sources.
How to train your synchrotron
Machine learning fundamentally requires two things: a reproducible problem and huge amounts of data. In this study, researchers fed electron-beam data from the ALS, including the positions of the IDs and the blips in electron-beam performance caused by ID adjustments, into a neural network. The algorithm was then able to learn the complex nonlinear relationships between the ID settings and vertical beam size and make corrections to negate the blips. The vertical beam size was stabilized to within 0.2 µm, or 0.4% of the beam size, compared to 2–3% without correction. The system is also fast: it can make corrections up to 10 times per second, although three times per second appears to be adequate for improving performance at this stage.
One key advantage of this approach is that data acquisition for retraining the neural network can be carried out continuously, even while the feed-forward system is active during a regular user run. Continuous retraining allows the neural network to constantly adapt to a drifting machine and changes in ID configurations during run periods, independent of static physics models.
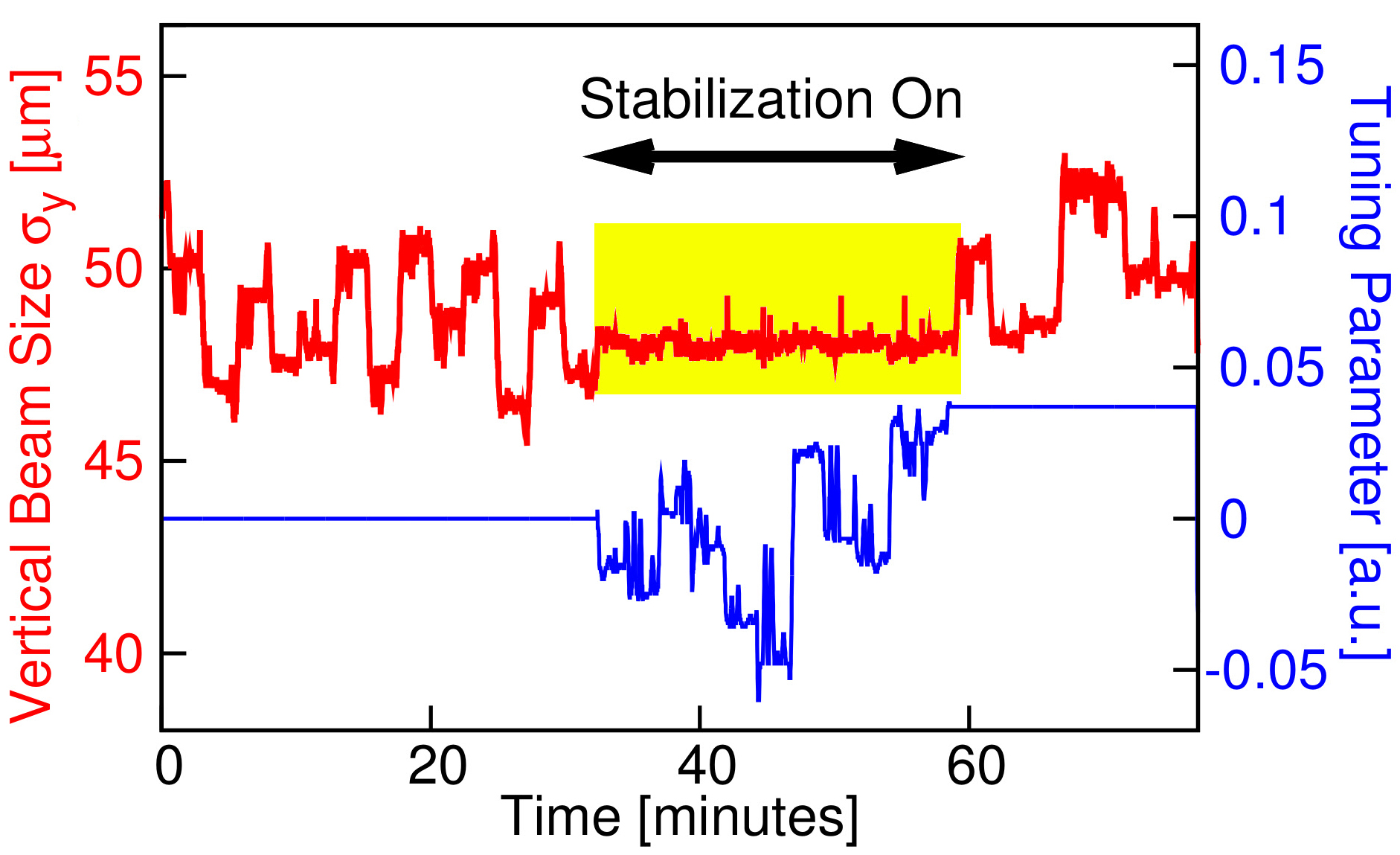
Future-ready, steady light beams
Because the size of the electron beam mirrors the resulting light beam, this correction scheme also optimizes the light reaching beamline endstations. To demonstrate this, the researchers observed the effects of the algorithm on scanning transmission x-ray microscopy (STXM) images taken at Beamline 5.3.2.2. Highly sensitive to low-frequency variations in light intensity, STXM scans will exhibit banding in response to ID motion elsewhere in the ring. With the neural-network correction on, the bands disappeared.
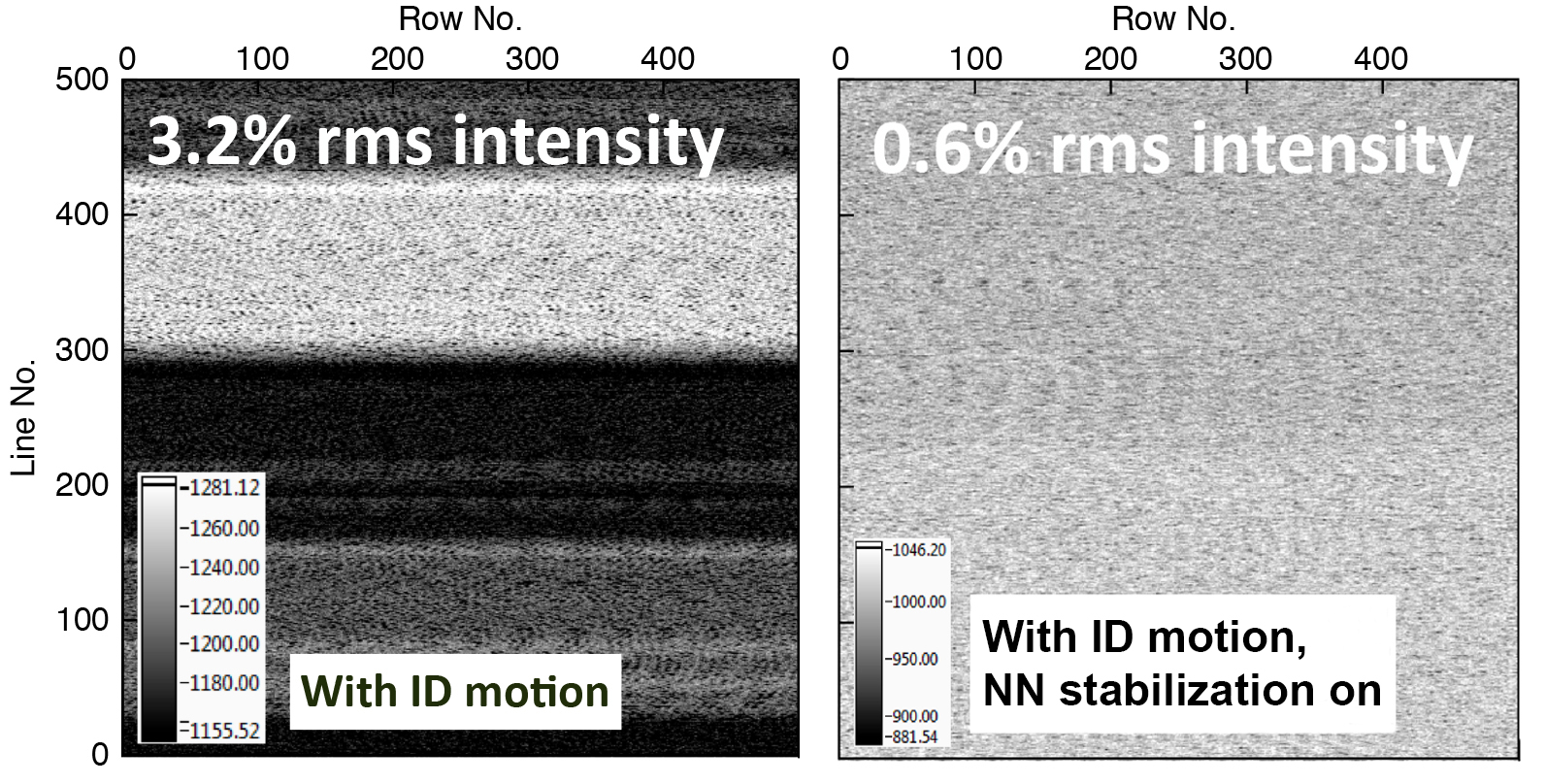
This enhanced performance is also well suited for advanced x-ray techniques such as ptychography, which can resolve the structure of samples down to the level of nanometers, and x-ray photon correlation spectroscopy (XPCS), which is useful for studying rapid changes in highly concentrated materials that don’t have a uniform structure. The technique could also generally be applied to other light sources and will be especially beneficial for specialized studies enabled by the ALS Upgrade (ALS-U) Project, which will also create a higher demand for consistent, reliable light-beam properties.
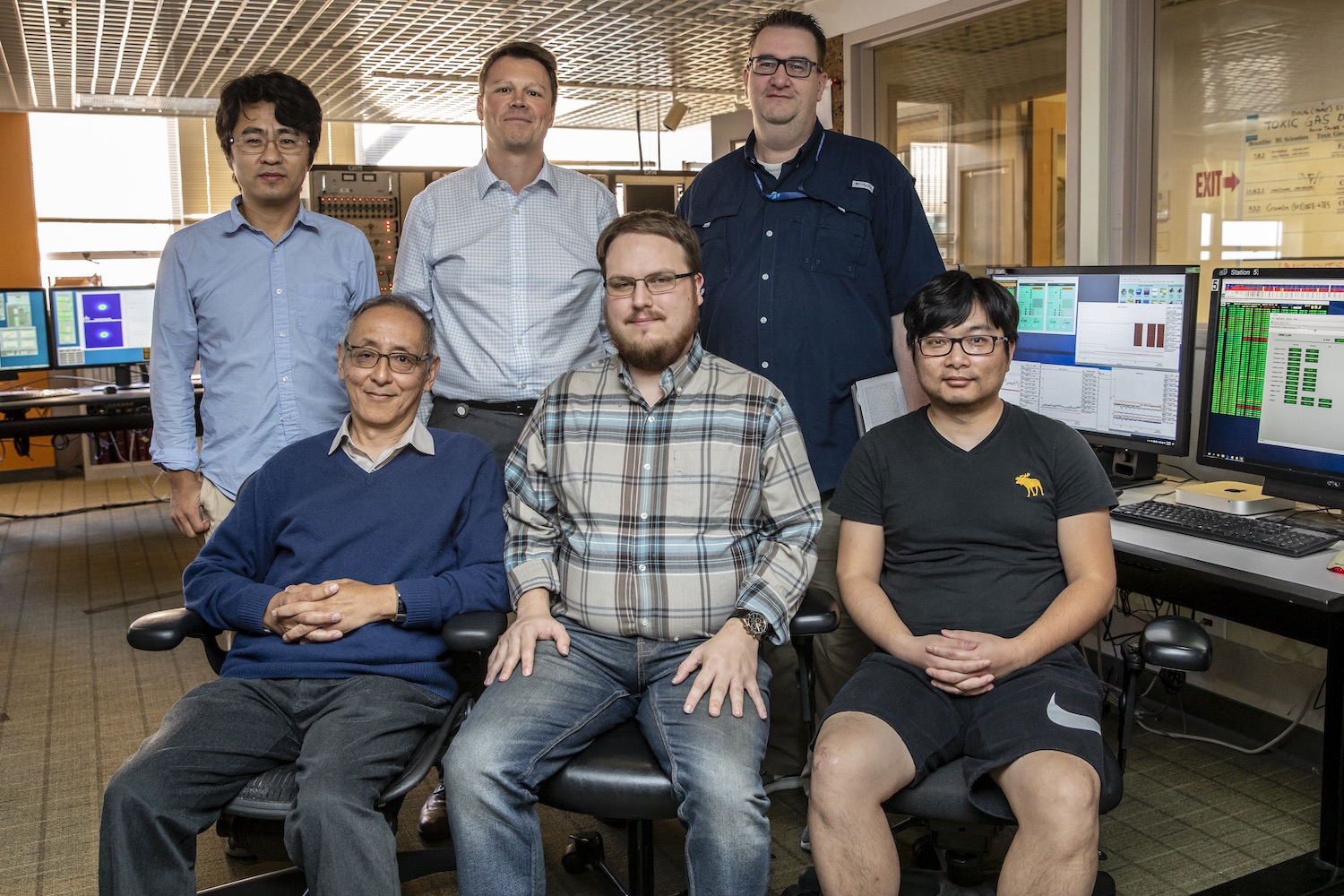
Contact: Simon Leemann
Researchers: S.C. Leemann, H. Nishimura, and C. Sun. (Berkeley Lab); S. Liu (University of California, Berkeley); and A. Hexemer, M.A. Marcus, and C.N. Melton (ALS).
Funding: U.S. Department of Energy (DOE), Office of Science, Advanced Scientific Computing Research (ASCR) and Basic Energy Sciences (BES) Programs. Operation of the ALS is supported by DOE BES.
Publication: S.C. Leemann, S. Liu, A. Hexemer, M.A. Marcus, C.N. Melton, H. Nishimura, and C. Sun, “Demonstration of machine learning-based model-independent stabilization of source properties in synchrotron light sources,” Phys. Rev. Lett. 123, 194801 (2019), doi:10.1103/PhysRevLett.123.194801.
Adapted from the Berkeley Lab news release, “Machine Learning Enhances Light-Beam Performance at the Advanced Light Source.”
ALS SCIENCE HIGHLIGHT #408