The ever-increasing quantity of data collected at scientific user facilities offers the opportunity to glean unprecedented interdisciplinary insights. To take full advantage of the wealth of information, researchers are turning to artificial intelligence (AI) and machine learning (ML) to pose and answer complex scientific questions. The U.S. Department of Energy (DOE) Basic Energy Sciences (BES) program recently awarded a combined $8.55 million to two Berkeley Lab-led teams for projects “aimed at both automating facility operations and managing data modeling, acquisition, mining, and analysis for the interpretation of experimental results.” In the press release announcing the awards, Chris Fall, Director of DOE’s Office of Science said, “These awards will help ensure America remains on the cutting edge of these critical technologies for science.”
MLExchange: A shared platform to discover new science from user facility data
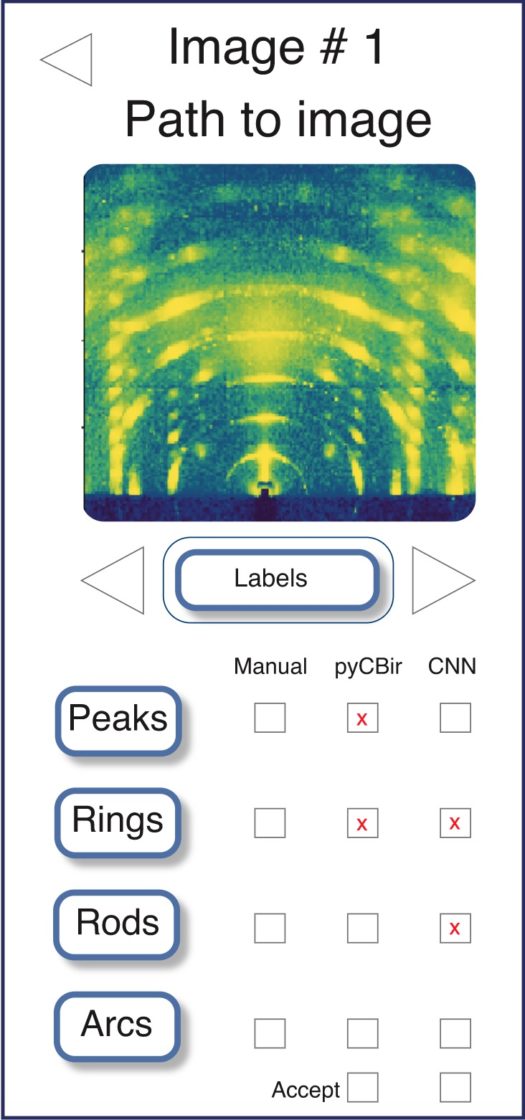
At the Advanced Light Source (ALS), Computing Program Lead Alex Hexemer saw that users needed improved tools to interpret their data. In July 2019, BES funded the Data Solution Task Force pilot, which brought together scientists from five light sources to start standardizing beamline controls, data description, and analysis tools across the DOE complex. CAMERA at Berkeley Lab developed the software program Xi-CAM, which is a versatile graphical user interface for visualization, data collection, workflow design, and data analysis, and has already been deployed at other light sources. The ALS has also started implementing software from Brookhaven National Laboratory’s NSLS-II light source. The data solution task force pilot combines these developments with additional software from SLAC National Accelerator Laboratory and Argonne National Laboratory. “We don’t have to reinvent the wheel,” said Hexemer. “There’s a lot of coordination necessary, but it’s pretty exciting to us.” Beyond the immediate benefits to users, the coordinated software and collaboration arising from the data pilot provide the infrastructure to support grander projects.
Now, the DOE has awarded $4.275 million over three years for a new data tool, MLExchange. MLExchange is a shared platform that lowers the barrier to entry by leveraging advances in ML methods across user facilities, thus empowering domain scientists and data scientists to discover new information using existing and new data with novel tools. Hexemer will lead a team comprising experimental, computational, and theoretical researchers at Berkeley Lab, which is allocated about half of the award amount, and at four other national laboratories—Argonne, SLAC, Oak Ridge, and Brookhaven. Although ML has taken root in many industries, Hexemer noted, “The availability of AI and ML tools in synchrotrons is pretty spotty. It takes time and investment, and if you don’t know if it works, are you going to make that effort?” MLExchange will make that effort on behalf of synchrotron scientists. The first project goal is to build a database, Splash ML, for storing and retrieving labels, which is already under development by ALS Computer Systems Engineer Dylan McReynolds. The next steps will involve further buildout of ML tools for users to manage their data.
“There’s a wealth of information you could have from people who already labeled data,” Hexemer said, providing a vision of datasets and models that are continually trained and improved. “MLExchange will help the researcher at the beamline and later with data analysis.”
4DCamera Distillery: From massive electron microscopy scattering data to useful information with AI/ML
At the Molecular Foundry, the National Center for Electron Microscopy (NCEM) has long been a leader in electron-optical characterization of materials, hosting a suite of state-of-the-art instrumentation and world-class expertise. Last year, a team of researchers introduced the fastest electron detector ever made. Known as the “4D Camera” (for Dynamic Diffraction Direct Detector), the detector can generate a daunting 4 terabytes of data per minute at 87 kHz. The massive data velocities and volumes present a significant challenge for moving, storing, and processing information.
Now, a team led by Andrew Minor, Director of NCEM, was awarded $4.275 million over three years by DOE to develop the 4DCamera Distillery, a program that will develop and deploy methods and tools based on AI and ML to analyze electron scattering information from the data streams of fast direct electron detectors. The team behind the effort, composed of researchers from Brookhaven National Laboratory, Oak Ridge National Laboratory, Argonne National Laboratory, Sandia National Laboratory, and Los Alamos National Laboratory, as well as Berkeley Lab, will address both the critical need for data reduction tools for these detectors and capitalize on the scientific opportunities to create new modes of measurement and experimentation that are enabled by fast electron detection.
“The 4DCamera Distillery combines expertise in electron microscopy experimentation, detector technology, and leading-edge AI/ML researchers,” said Minor. “It will enable materials characterization that is in high demand by the DOE Nanoscience Research Center user community.”