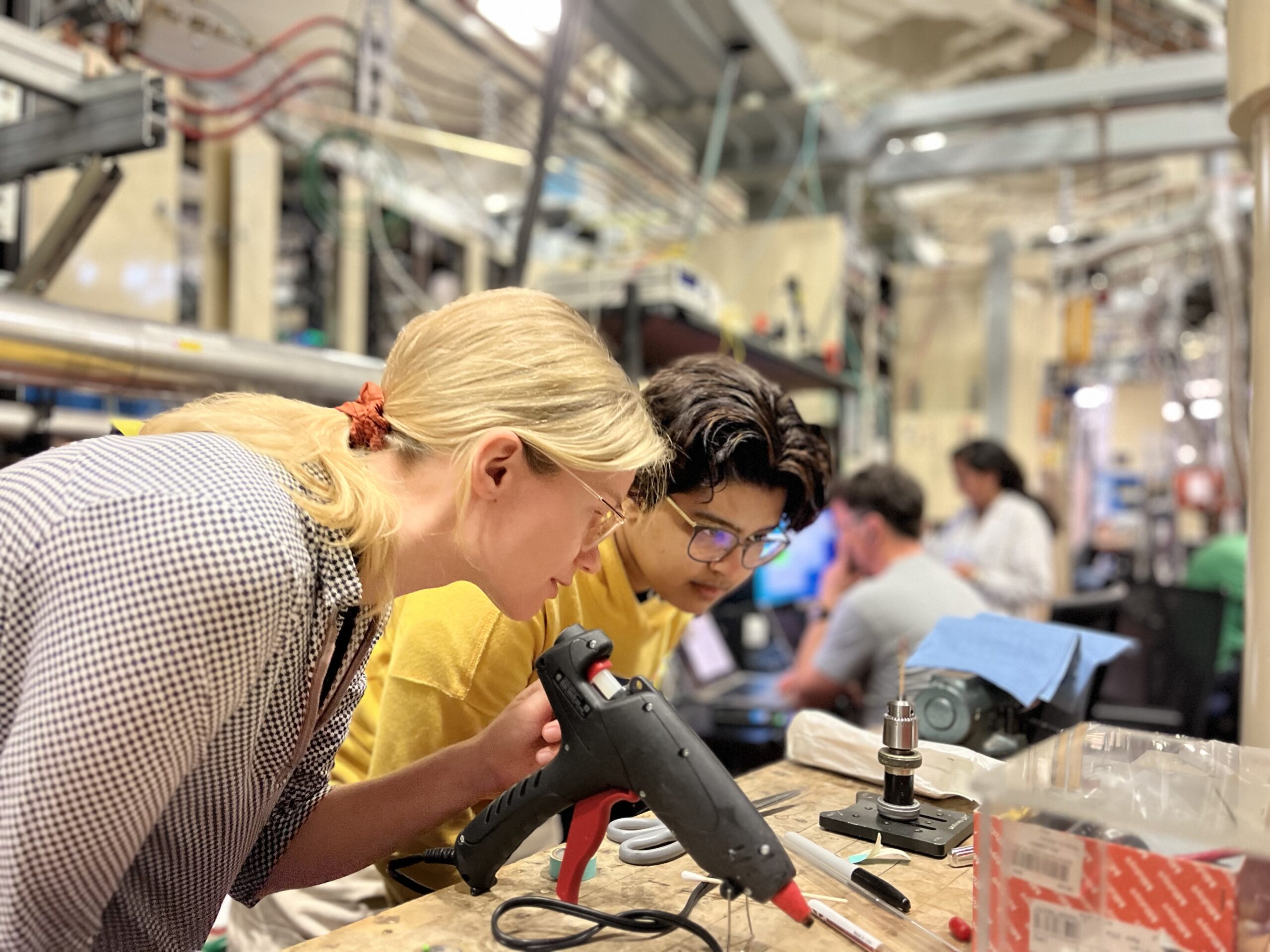
From the types of samples to the techniques used to study them, user experiences at beamlines around the world can vary, but one commonality connects them: beamtime is precious. At different facilities, users encounter different beamline controls, and varying availability of compute infrastructure to process their data. Beyond needing to familiarize themselves with different equipment and software setups, they also need to ensure that they’re collecting meaningful, consistent data no matter where they are. For the past several months, the ALS Computing group has been traveling around the world for beamtime. Their firsthand experience is informing the development of a suite of tools aimed at lowering the barriers of access to advanced data processing for all users.
Today’s beamtime experience
As a beamline scientist at the ALS, Dula Parkinson has helped numerous users with microtomography, a technique that can yield ten gigabytes of data in two seconds. “In many cases, users won’t have done this kind of experiment or analysis before, and they won’t have the computing infrastructure or software needed to analyze the huge amounts of complex data being produced,” he said.
Computational tools and machine-learning models can help the users, from adjusting their experimental setup in real time to processing the data after the experiment has concluded. Eliminating these bottlenecks can make the limited beamtime more efficient and help users glean scientific insights more quickly.
As a former beamline scientist himself, Computing Program Lead Alex Hexemer has first-hand knowledge of the user experience. He was instrumental in the creation of a dedicated computing group at the ALS in 2018, which continues to grow in both staff numbers and diversity of expertise. A current focus for the group is to advance the user experience with intuitive interfaces.
Computing approach to beamtime
Recently, Hexemer and two of his group members, Wiebke Koepp and Dylan McReynolds, traveled to Diamond Light Source, where they worked with Beamline Scientist Sharif Ahmed to test some of their tools during a beamline experiment. “It is always useful to see other facilities from the user’s perspective,” McReynolds said. “We want our software to be usable at many facilities, so getting to test in other environments was very valuable.”
The computational infrastructure is an essential complement to the beamline instrumentation. To standardize their experiments across different microtomography beamlines, the team performed measurements on a reference material—sand with standardized size distributions. Each scan captures a “slice” from the sample; the slices then need to be reconstructed into three-dimensional images that contain 50 to 200 gigabytes of data.
Within that data, the researchers need to glean meaningful information. “We need to segment the data,” explained Hexemer. “This is sand. This is the vial holding the sand. This is air in between.” Identifying the segments allows researchers to more easily decide where to take the next scan—in essence where to move the beam to detect more sand and less vial. But, this type of analysis has traditionally happened after an experiment. That means that researchers might take more scans than necessary, because some scan parameters yield less insightful measurements.
Here, the computing group saw a need for users to assess the quality of their data in near-real-time. “The goal is to be able, at the moment when a scan comes in, to do some immediate analysis to inform the experiment further,” Koepp said. “Our goal is that, algorithmically, you’ll be able to greatly reduce the number of scans you need to take to get the same amount of meaningful data,” McReynolds added.
The seed for this idea has been planted; Berkeley Lab Staff Scientist Peter Zwart and his collaborators in CAMERA developed machine learning algorithms for segmentation. Through beamtime at Diamond and the ALS, the computing group is expanding the functionality and testing the robustness of the algorithms. “We’re replicating the experimental setup at different facilities as closely as possible,” Koepp said, “because different data processing steps, different exposure times, etc., could all potentially affect model performance.”
But, to take advantage of these algorithms, synchrotron users need to be able to access and use powerful computational infrastructure that can parse the many gigabytes, and even terabytes, of data.
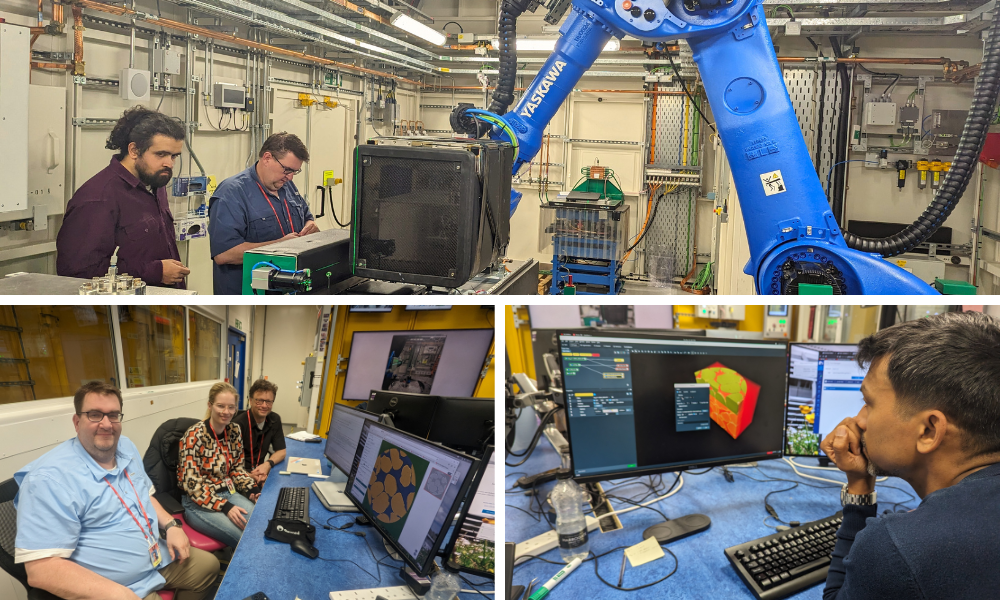
User-friendly (and user-facility-friendly) computing for the future
The ALS can facilitate user access to computational infrastructure, like the NERSC supercomputing facility. But, the users still need a portal into NERSC and a simple interface that doesn’t require a background in coding.
The Computing group is addressing this need by developing a web interface for users as part of the MLExchange project. “We’re trying to give users access to fantastic hardware in web interfaces that are easy to use,” said Hexemer. “When they come for such a short beamtime, they won’t have to write code just to use the computational infrastructure,” he added.
McReynolds expanded upon the goals for the user experience. “We want to make it easy for the algorithm to interface with different beamline hardware,” he said. And so, after testing their tools at the DIAD beamline at Diamond Light Source with Ahmed, the Computing group returned to the ALS to perform the same scans on the same samples at Beamline 8.3.2 with Parkinson to test the robustness of their machine-learning model.
The machine-learning models hold great potential to facilitate cross-facility learning, enabling more-efficient experiments. “If somebody scans sand and trains the network, somebody at another facility could use the same model to segment their sand, or maybe just fine tune their analysis instead of starting from scratch,” Hexemer explained.
The cross-facility learning is not limited to the machine learning models. In fact, these advances are made possible by people around the world all contributing different insights and experiences. The ALS Computing team, including Hexemer, Koepp, and McReynolds, as well as Tanny Chavez, Tibbers Hao, Raja Vyshnavi Sriramoju, and Xiaoya Chong, has been collaborating with Zwart at Berkeley Lab, Tim Snow and Jacob Filik at Diamond, and beamline scientists at ALS Beamlines 8.3.2 and 7.3.3, Diamond, and DESY. Much of their work is part of the MLExchange, which is a collaboration Hexemer leads with user facilities at SLAC, Oak Ridge, Argonne, and Brookhaven National Laboratories. This type of cross-facility learning delivers cross-facility results. “We want to make sure that all the pipelines we build can be easily taken somewhere else and used at other beamlines,” said Hexemer.
The ALS Computing group’s web interface will provide synchrotron users with real-time feedback and analysis capabilities at different beamlines around the world. Starting as a tool to resolve experimental bottlenecks, computing is evolving to become an essential building block for the experimental framework itself. In fact, Parkinson can already envision applications on a grand scale. “They’re taking a serious step toward providing a ‘digital twin’ of the sample, allowing users to really understand and simulate their experiments,” he said. With synchrotron data feeding into a machine-learning model, and a machine-learning model guiding data collection, all accessible to the end user, the future of synchrotron science is poised to answer questions at the limits of our imagination.
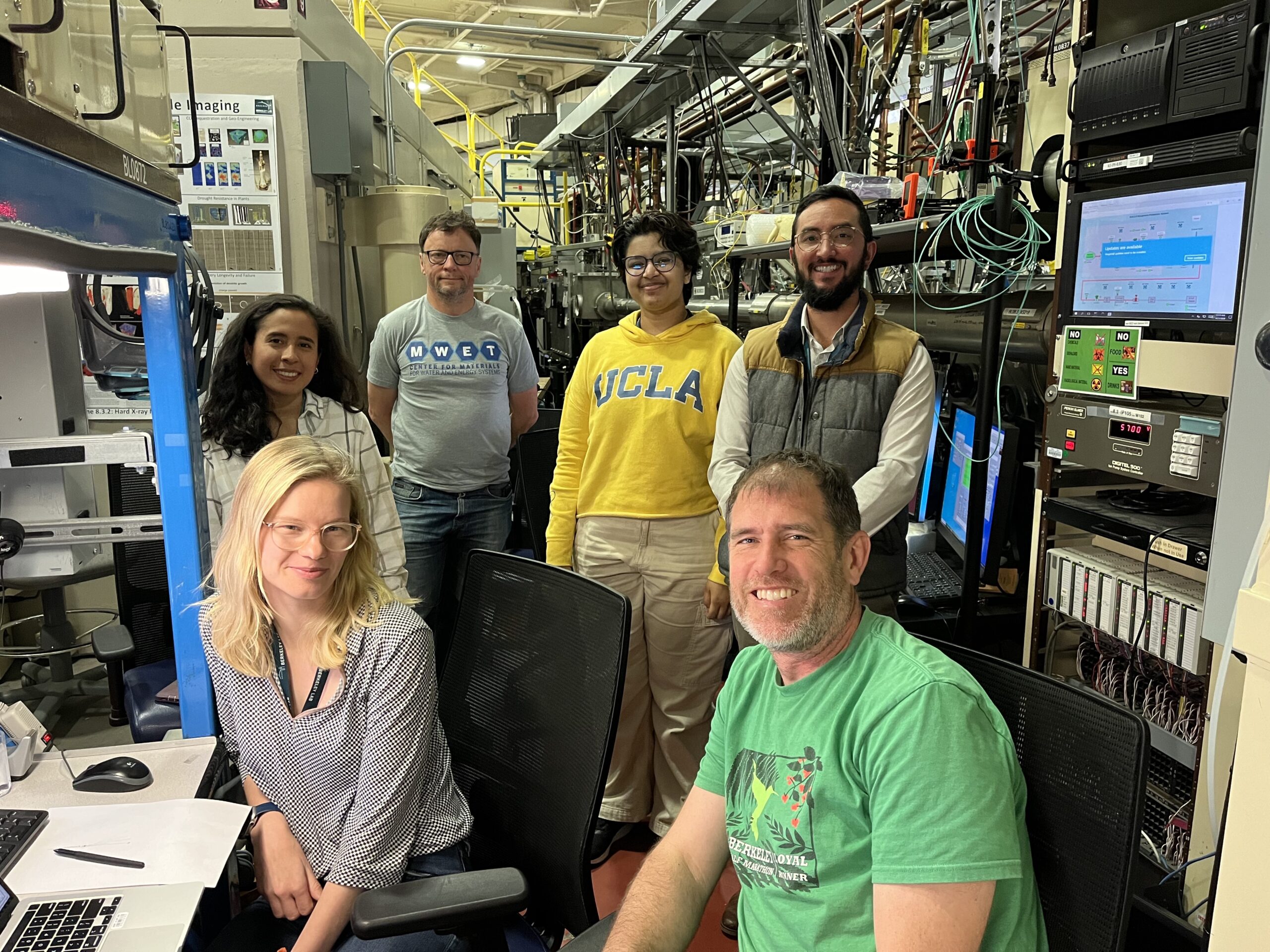