SCIENTIFIC ACHIEVEMENT
Researchers at the Advanced Light Source (ALS) and Advanced Photon Source (APS) used machine learning to predict and control the shape of an x-ray mirror’s surface with exquisite accuracy and precision.
SIGNIFICANCE AND IMPACT
The work represents a key step toward mirrors that can fully exploit the x-rays from upgraded, state-of-the-art light sources and could also enable the engineering of x-ray beams with novel characteristics.
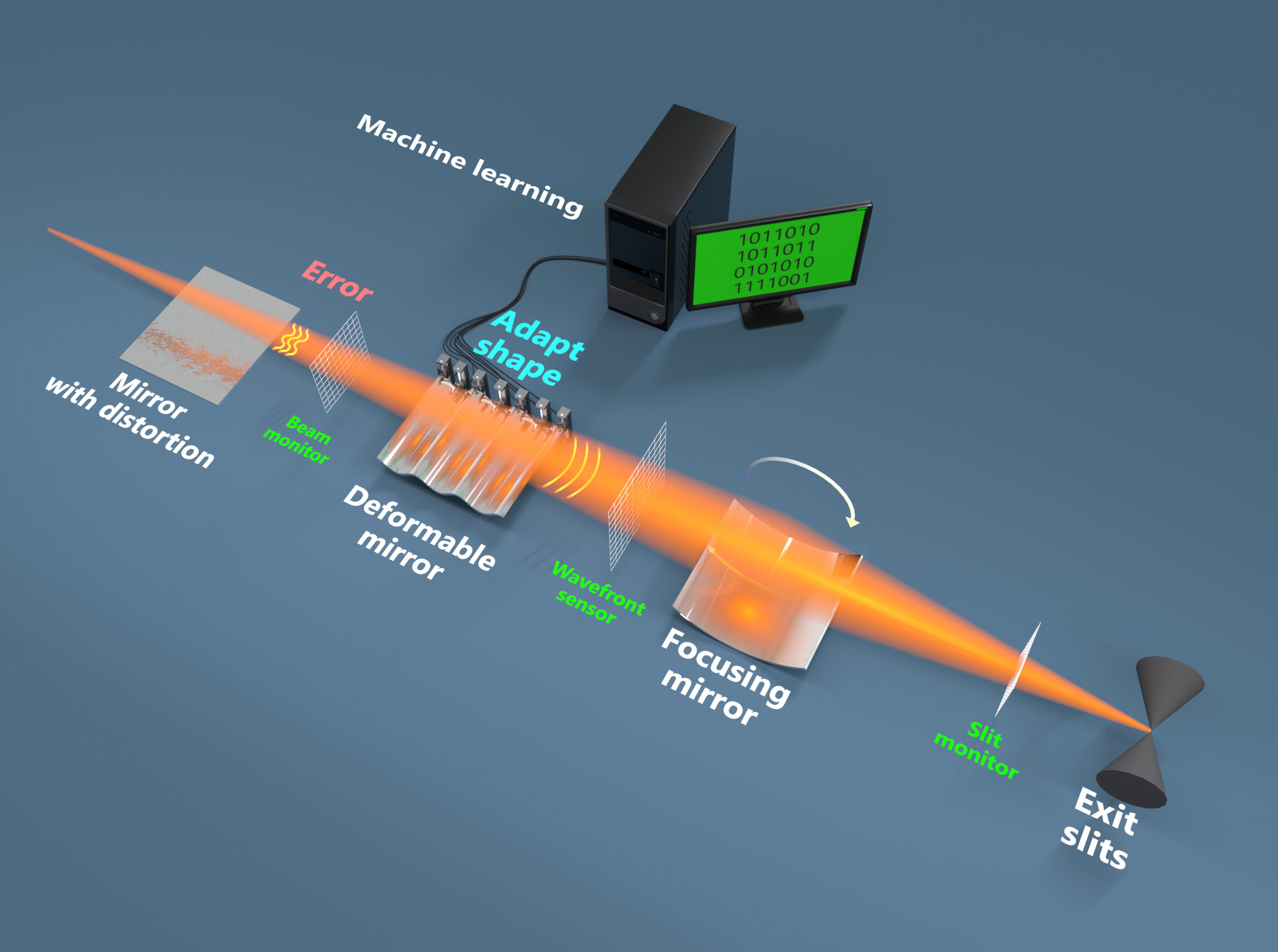
Shaping the future of x-ray light
To fully benefit from major upgrades that are just around the bend for x-ray light sources such as the ALS at Berkeley Lab and the Advanced Photon Source (APS) at Argonne National Laboratory, it’s important to also optimize supporting systems as well. For example, mirrors that direct x-rays through a beamline can introduce errors into an otherwise perfect x-ray wavefront, limiting the quality of experimental results.
Adaptive optics, in which a mirror’s surface is adjusted to compensate for errors using piezoelectric materials that expand or contract in response to an applied voltage, have been used in infrared and visible-light astronomy for decades. However, for synchrotrons operating in the x-ray regime, where wavelengths are a hundred times smaller, the tolerances for error are a hundred times tighter.
In this collaboration between the ALS and APS, researchers used machine learning to predict and control the shape of a deformable x-ray mirror, in real time and without the need for continuous feedback.
Fixing errors in x-ray mirrors
The concentrated energy from a storage-ring x-ray beam directed onto a mirror—typically made of platinum-coated silicon—can cause deformations exceeding tolerances for next-generation light sources. While adaptive mirrors could be a solution, their adoption at x-ray beamlines must account for complicating effects such as cross-talk between actuators, nonreversible movements, and continued displacement after the voltage stops changing.
Furthermore, adaptive mirrors typically require feedback to respond in real time. In the case of an x-ray beamline, this would mean interrupting the beam to measure it, which is not ideal for experiments and is difficult to do with the required precision. However, recent success in applying machine-learning methods to stabilize the electron beam in the ALS storage ring has inspired a similar approach to optimizing adaptive x-ray mirrors.
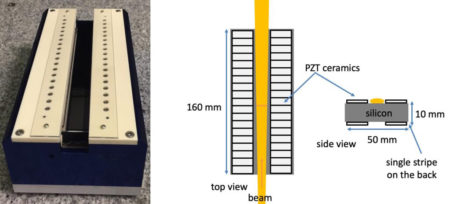
Data-driven machine learning
In this work, researchers trained a neural network using data that related a mirror’s shape to random voltage inputs. Then, the neural network’s ability to predict the mirror’s shape, given different voltage inputs, was tested. The results showed that the program produced fewer prediction errors than a simple linear response model. The researchers also demonstrated the program’s ability to direct the mirror to achieve and hold arbitrary shapes, with excellent results.
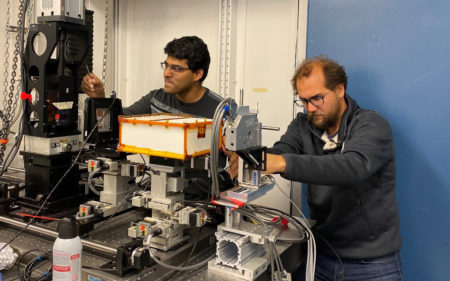
The experiments were performed at APS, with the machine-learning work done at the ALS by UC Berkeley graduate student Gautam Gunjala under a Department of Energy (DOE) Office of Science Graduate Student Research (SCGSR) award. The results are also relevant to the work of ALS research scientist and co-author Antoine Wojdyla, as a starting point for his project on Diffraction-limited Radiation Enhancement with Adaptive Mirrors (DREAM beams), funded by the DOE Early Career Research Program. One goal of the project is to “wavefront engineer” x-ray beams to enable higher experimental sensitivities and promote newly emerging imaging modes (e.g., holographic interference and donut-shaped beams).
Work is already underway to show that this machine-learning approach works in an operating beamline. Eventually, data-driven modeling and control of adaptive mirrors will be used at all state-of-the-art light sources such as the upgraded ALS, to obtain the best possible performance and take full advantage of the beam’s post-upgrade coherence.

Contact: Antoine Wojdyla
Researchers: G. Gunjala (UC Berkeley, ALS, and APS); A. Wojdyla and K.A. Goldberg (ALS); Z. Qiao, X. Shi, and L. Assoufid (APS); and L. Waller (UC Berkeley).
Funding: U.S. Department of Energy (DOE), Office of Science Graduate Student Research program. Operation of the ALS and APS is supported by DOE Office of Science, Basic Energy Sciences (BES) program.
Publication: G. Gunjala, A. Wojdyla, K.A. Goldberg, Z. Qiao, X. Shi, L. Assoufid, and L. Waller, “Data-driven modeling and control of an x-ray bimorph adaptive mirror,” J. Synchrotron Radiat. 30, 57 (2023), doi:10.1107/S1600577522011080.
ALS SCIENCE HIGHLIGHT #478