SCIENTIFIC ACHIEVEMENT
Researchers extracted the relationship between strain and composition in a battery material by applying machine-learning methods to atomic-scale images obtained in part at the Advanced Light Source (ALS).
SIGNIFICANCE AND IMPACT
The work could lead to more durable batteries and also highlights the potential of integrating microscopy techniques with machine learning to gain insights into complex materials.
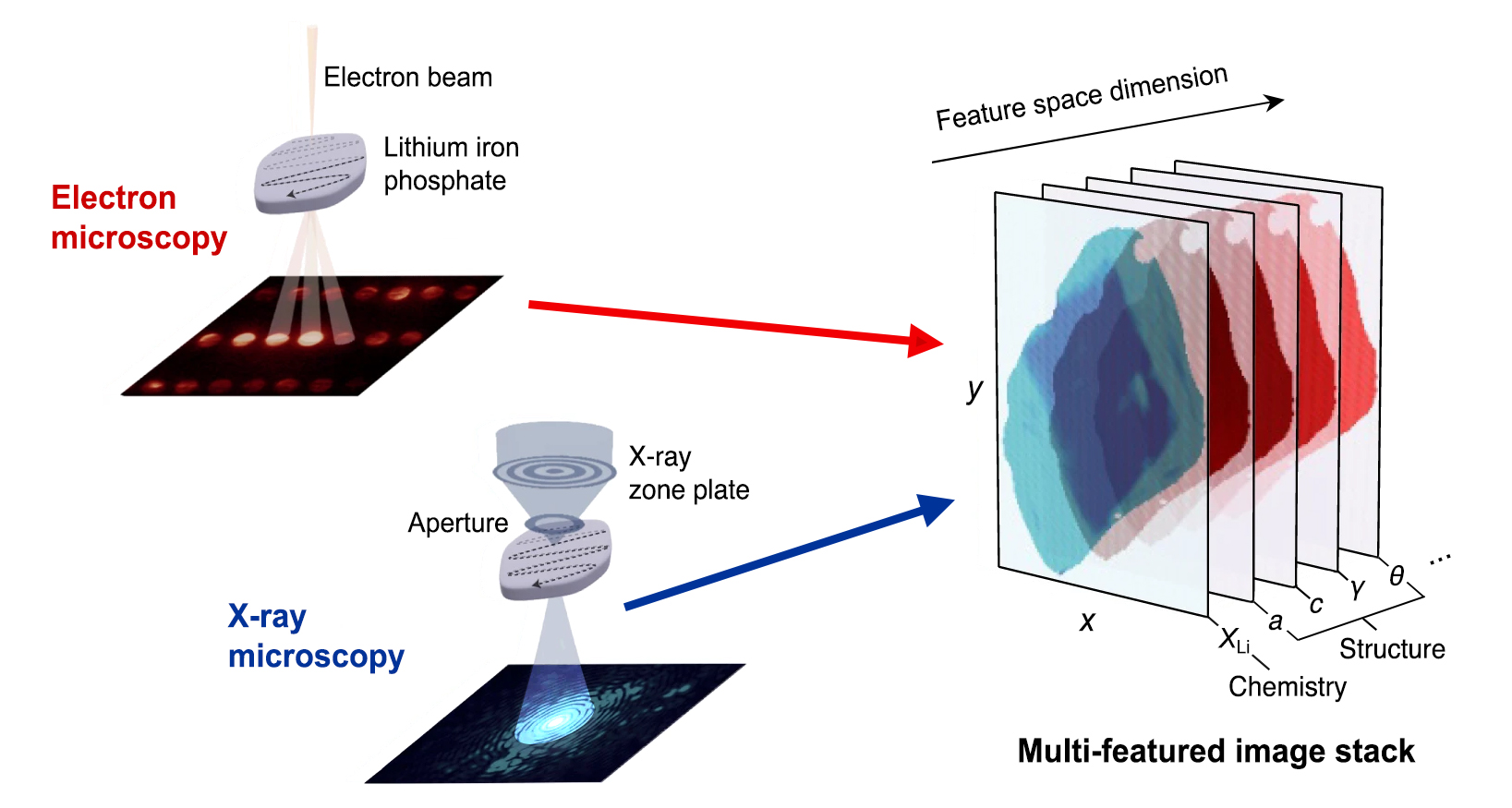
Science in charge of charging
Today’s rechargeable batteries are a wonder, but they’re still far from perfect. Eventually, they wear out and need recycling and (sometimes expensive) replacement. But what if batteries were indestructible? A new analytical approach to building better batteries could help speed the arrival of that day.
Researchers from Stanford University and collaborators at Berkeley Lab, MIT, and other institutions recently developed a machine-learning method to quantitatively analyze high-resolution microscopy images from a battery cathode material. Their image-learning framework was able to extract the underlying physical relationship between strain and composition, a fundamental material property relevant to battery failure. Eventually, the researchers say, the revelations could lead to batteries that last much longer than today’s.
The nanofracture factor
A material’s performance is a function of both its chemistry and the physical interactions occurring at the atomic scale, what scientists refer to as “chemo-mechanics.” What’s more, the smaller things get and the more diverse the atoms making up the material are, the harder it is to predict how the material will behave.
For example, the cathode in a rechargeable battery is the source of the lithium ions that flow back and forth during each charge–discharge cycle. The mechanical strain on the cathode material eventually results in nanofractures, leading to battery failure.
Lithium iron phosphate (LixFePO4, or LFP) is a cathode material that’s gaining popularity with electric car makers and other battery-intensive businesses. However, key questions remain unanswered regarding the material’s elasticity and deformation, particularly when lithium-rich and lithium-poor phases are present simultaneously.
Correlative image learning
In this work, the researchers combined two complementary, high-resolution microscopy techniques. First, a new kind of scanning transmission electron microscopy (4D-STEM), performed at Berkeley Lab’s Molecular Foundry, provided information about localized strains in a given particle by measuring lattice deformations. Second, x-ray spectro-ptychography, performed on the same particles at ALS Beamline 7.0.1.2 (COSMIC), provided nanoscale-resolution, element-specific maps of lithium composition. The images were aligned into stacks containing each particle’s correlated compositional and structural features.
To extract the connection between composition and strain, the researchers applied a machine-learning algorithm that took into consideration the data, theoretical models, and physical constraints. The results showed that the strain varies almost linearly with lithium composition—a direct, nanoscale validation of Vegard’s law for alloys, an empirically derived relationship between an alloy’s lattice constant and its elemental concentration.
New directions for the future
The researchers note that, in addition to being relevant to improving battery lifetimes, their method is generalizable to most crystalline materials, particularly those that are difficult to characterize. They also anticipate that the approach can be adapted to dynamic systems, where laws governing reaction kinetics and transport can be learned.
One practical outcome might be battery-control software that manages charging and discharging to improve battery life. Another is the development of more accurate computational models that allow engineers to explore alternative electrode materials on a computer.
In general, this image-learning framework provides both the means and motivation to develop a more fundamental understanding of the mechanics at play in functional materials, demonstrating the potential of merging correlative microscopy with physics-constrained image learning for scientific discovery.
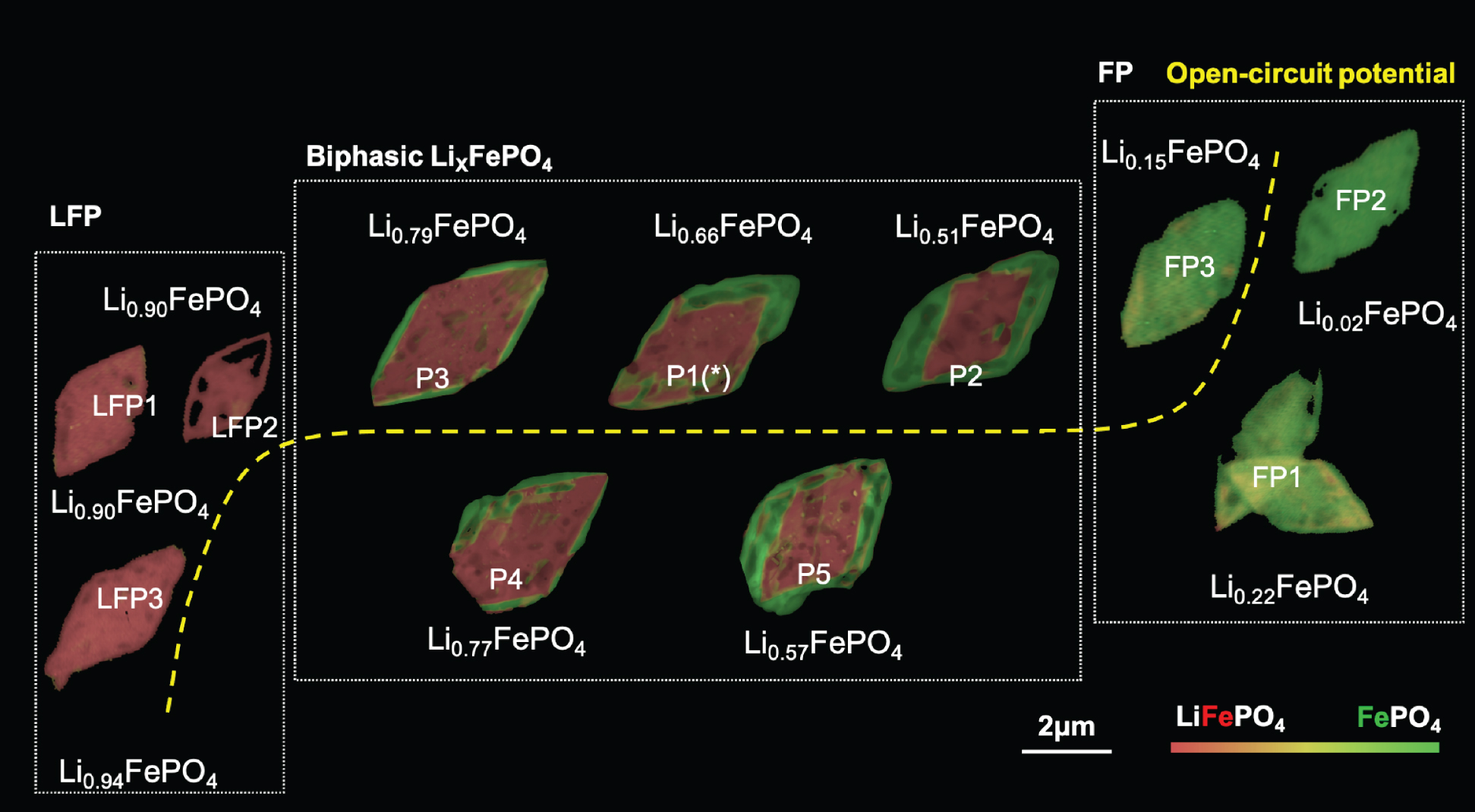
Contact: Will Chueh
Researchers: H.D. Deng, N. Jin, E.G. Lomeli, R. Yan, J. Liu, and W. Cai (Stanford University); H. Zhao, D. Fraggedakis, and M.Z. Bazant (Massachusetts Institute of Technology); L. Hughes, B.H. Savitzky, and C. Ophus (Berkeley Lab); A. Borbély (Mines Saint-Etienne, France); Y.-S. Yu (ALS and Chungbuk National University, Republic of Korea); D.A. Shapiro (ALS); A.M. Minor (Berkeley Lab and UC Berkeley); and W.C. Chueh (Stanford University and SLAC National Accelerator Laboratory).
Funding: Toyota Research Institute, National Science Foundation, and U.S. Department of Energy, Office of Science, Basic Energy Sciences program (DOE BES). Operation of the ALS is supported by DOE BES.
Publication: H.D. Deng, H. Zhao, N. Jin, L. Hughes, B.H. Savitzky, C. Ophus, D. Fraggedakis, A. Borbély, Y.-S. Yu, E.G. Lomeli, R. Yan, J. Liu, D.A. Shapiro, W. Cai, M.Z. Bazant, A.M. Minor, and W.C. Chueh, “Correlative image learning of chemo-mechanics in phase-transforming solids,” Nat. Mater. 21, 547 (2022), doi:10.1038/s41563-021-01191-0.
Adapted from the Stanford University press release, “Stanford scientists combine AI and atomic-scale images in pursuit of better batteries.”
ALS SCIENCE HIGHLIGHT #463