Traditional artificial intelligence (AI) hardware employs physically separated information sensing, processing, and memory architecture, a configuration that suffers from large energy and time overhead due to frequent data shuttling between hardware modules and the need for sequential analog-to-digital conversion. In this work, researchers report the fabrication of an organic electrochemical transistor (OECT) device that can act as both the sensor and processing core of a streamlined AI hardware system.
“Briefly, we fabricated a device that can simultaneously work like the CPU and memory in your PC, and it can also work like a sensor on your smart watch,” said Wei Ma, a professor of materials science and engineering at Xi’an Jiaotong University. “The integration of the various devices is promising for smart computing tasks, with higher efficiency.”
The researchers synthesized an organic material (PTBT-P) that conducts both ions and electrons and, upon annealing, includes both crystalline and amorphous regions. Grazing-incidence wide-angle x-ray scattering (GIWAXS) at Advanced Light Source Beamline 7.3.3 was used to track the behavior of ions injected into the material. The resulting scattering profiles revealed a peak whose displacement was reversible at high and low doping potentials, indicating that the ions are trapped in the crystalline region and de-trap only when a sufficient positive potential is applied.
This structural tunability enables both volatile and nonvolatile behavior, depending on applied voltage. The volatile sensing behavior allows the material to be responsive to stimuli such as ions and light. The nonvolatility allows it to store and process 10-bit analog states. This versatility is highly desirable in neuromorphic circuits—i.e., circuits inspired by biological nervous systems, which outperform artificial neural networks based on conventional silicon hardware.
As proof of concept, the researchers used PTBT-P transistors to sense ion concentration changes in plants and record electrocardiogram signals. They also explored, via simulations, the potential use of the integrated devices in real-time cardiac disease diagnoses. In the long term, the technology could be key to the development of edge AI, where localized devices at the “edge” of a network are able to learn from data collected in situ, without the need for connecting to a centralized processor.
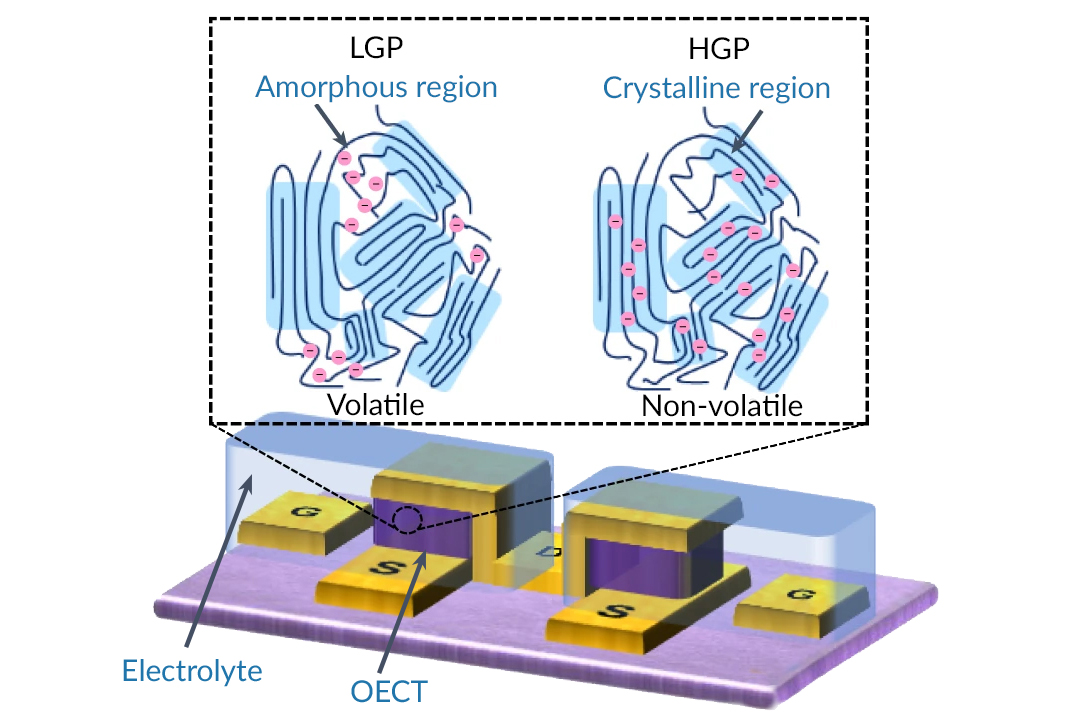
S. Wang, X. Chen, C. Zhao, Y. Kong, B. Lin, Y. Wu, Z. Bi, Z. Xuan, T. Li, Y. Li, W. Zhang, E. Ma, Z. Wang, and W. Ma, “An organic electrochemical transistor for multi-modal sensing, memory and processing,” Nat. Electron. 6, 281 (2023), doi:10.1038/s41928-023-00950-y.