by Lori Tamura
The topic of machine learning has been getting a lot of buzz lately—with good reason. At the Advanced Light Source (ALS), a team of accelerator physicists and computer scientists were able to use machine-learning techniques to solve a problem that has plagued third-generation light sources for a long time: fluctuations in beam size due to the motion of insertion devices.
“You look at the data and you realize, holy cow, this thing really just managed to reduce fluctuations by almost an order of magnitude!” said team leader Simon Leemann, deputy of the ALS Accelerator Physics Group. “That was the moment it really kicked in. That was quite special.” Alex Hexemer, a senior scientist who leads the Computing Program at the ALS, served as the co-lead in developing the new tool.
By improving the stability of the electron beam, the project successfully enhanced the performance of the ALS. As a result, Leemann, Hexemer, and fellow team members Shuai Liu, Yuping Lu, C. Nathan Melton, Hiroshi Nishimura, Changchun Sun, and Daniela Ushizima received the 2021 Klaus Halbach Award for Innovative Instrumentation at the ALS.
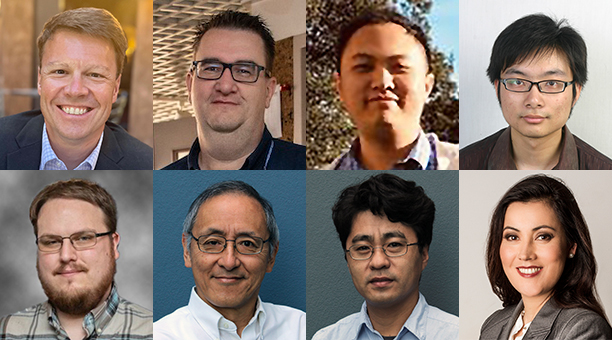
“Truly stunning results were achieved after just a few months of effort, and the approach is now codified and embedded in ALS accelerator operation,” wrote ALS Director Steve Kevan in support of the nomination. The experimental results have been described in a study published in Physical Review Letters and in a Berkeley Lab news release.
“Synchrotron facilities such as the ALS deliver light over a broad spectrum to dozens of simultaneous experiments,” said ALS Deputy for Accelerator Operations Fernando Sannibale, who nominated the team for the award. “Each straight-section beamline independently tunes the energy of the photons it delivers by adjusting the magnetic field generated by its insertion-device source.”
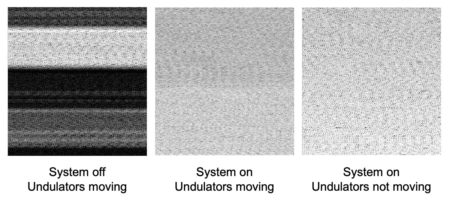
Such adjustments perturb the electron beam stored in the ALS ring and can affect the overall light-beam performance across the entire facility. In particular, the perturbations strongly and directly affect several classes of beamline experiments that extract the coherent fraction of photons emitted by the source, such as scanning transmission x-ray microscopy (STXM) at Beamline 5.3.2.2.
“Simply put, this will dramatically improve the quality of science our users can do,” said David Shapiro, who leads the Microscopy Program at the ALS. “I have no doubt that these methods will be implemented at all synchrotrons.”
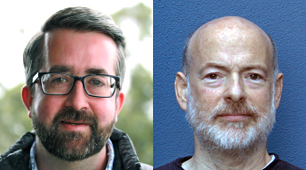
From the very beginning, Shapiro and ALS Staff Scientist Matthew Marcus were instrumental to the success of the project. They described the problem from the user perspective, devised measurement protocols, and gathered data during accelerator physics shifts, coordinating measurements with the control room. “None of this work would have been possible without their efforts and commitment,” said Leemann.
Changchun Sun, a staff scientist in the ALS Accelerator Physics Group, was also a key player on the team. “His measurement setup was absolutely critical to the success of this work,” said Leemann. “Without his expertise in imaging our beam and ability to tweak the instrument specifically for this application, we would not have been able to realize the project.”
Initially, Sun was skeptical that this scheme could really work. There are so many parameters to account for, and so many insertion devices, how could an algorithm accurately predict the beam’s fluctuations and then compensate for them in real time? It was Hiroshi Nishimura, an accelerator physicist at the ALS (now semi-retired), who helped turn the corner.
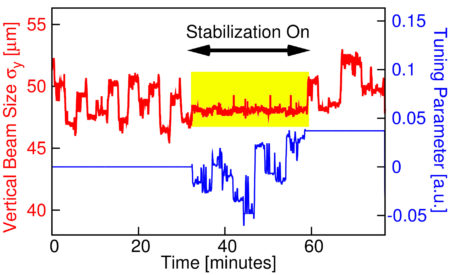
“When something got too complex for traditional methods to work with a reasonable amount of effort, I knew it was time to try machine learning,” said Nishimura. “Many textbook examples were demonstrating the usefulness of machine learning. There was no reason for it not to work like any other accelerator project on paper.”
Nishimura performed some preliminary tests, running historical data through a machine-learning program. The method’s predictive power astonished both Sun and Leemann. “Hiroshi made it work,” said Sun. “Hiroshi and the data, using machine learning, predicted the beam size! That was my turning point, when I knew that machine learning was going to be very useful.”
Nishimura’s interest in applying computer technology to accelerator physics led him to connect, through Hexemer, to Daniela (Dani) Ushizima, a data scientist in the Machine Learning and Analytics Group of Berkeley Lab’s Computational Research Division (CRD). In succession, CRD graduate student Shuai Liu (now at Facebook), ALS postdoc C. Nathan Melton (now at Lawrence Livermore National Laboratory), and postdoc Yuping Lu (currently wrapping up an appointment with the Accelerator Technology and Applied Physics Division) all contributed machine-learning and data-analysis expertise to the effort.
“This sort of work is emblematic of what is needed,” said Ushizima, “close cooperation between domain experts and data scientists, customizing and tailoring techniques to take advantage of scientific information to bring new levels of sophistication to machine-learning methods.”
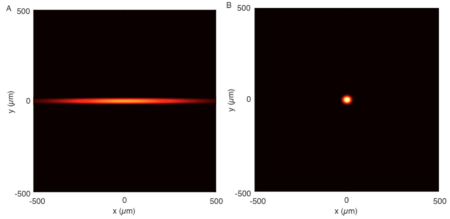
As the ALS Upgrade (ALS-U) project moves forward, such collaborations will continue to be crucial. The ALS-U beam will be very different—a point-like vs a “flat” beam. A flat beam is naturally very stable in the horizontal direction, but after the upgrade, stabilization will be needed in that direction as well. Team leader Leemann is already thinking about how to get it done.
“I’m convinced you can get it to work, but the way you implement the correction and the way you train this machine-learning model has to be adapted to work in both transverse planes,” he said. “It’s not going to be copy-and-paste. We’re going to actually have to develop it. It’s one of the things we want to do in a follow-on project—start developing those ideas.”
Klaus Halbach was a senior staff scientist at LBNL who pioneered the development of undulators using permanent magnets, and other innovations in accelerator physics. Even though he retired from LBNL in 1991, he remained active in lab projects and student training until his death in 2000.