Scientists have identified a way to use machine learning to integrate fine- and large-scale infrared characterizations of shale—sedimentary rocks composed of minerals and organic matter. The flow of fluids through shale’s nanoporous networks is fundamental to hydraulic fracturing and enhanced geothermal heating as well as to carbon sequestration and water storage. Thus, understanding shale chemistry at both the nano and mesoscale is relevant to energy production, climate-change mitigation, and sustainable water and land use.
The researchers first collected mesoscale chemical-composition maps hundreds of micrometers across using attenuated total reflection (ATR), an infrared technique that uses a hemispherical germanium crystal to achieve micrometer spatial resolution (Ge micro-ATR). At ALS Beamline 5.4, synchrotron infrared nanospectroscopy (SINS)—an infrared imaging technique based on infrared spectroscopy—was used to obtain similar maps with nanometer spatial resolution, but just a few micrometers in size.
The SINS measurements provided examples of cleanly resolved (“deconvoluted”) spectral signatures of the shale’s chemical constituents. This data was used to “train” a neural network to better interpret Ge micro-ATR data and predict the chemical composition with nanoscale accuracy over large areas. With this technique, the researchers found mineral phases that were co-located with chemically distinct organics, as well as evidence of micrometer-sized pockets of CO2 attached to clay surfaces, possibly even intercalated into the clay structure, a phenomenon that had been theorized, but not yet observed.
In principle, this cross-scale molecular analysis is not limited to infrared spectroscopy of shale rock; it could just as well be applied to many other applications such as hyperspectral remote sensing or live-cell imaging in the future.
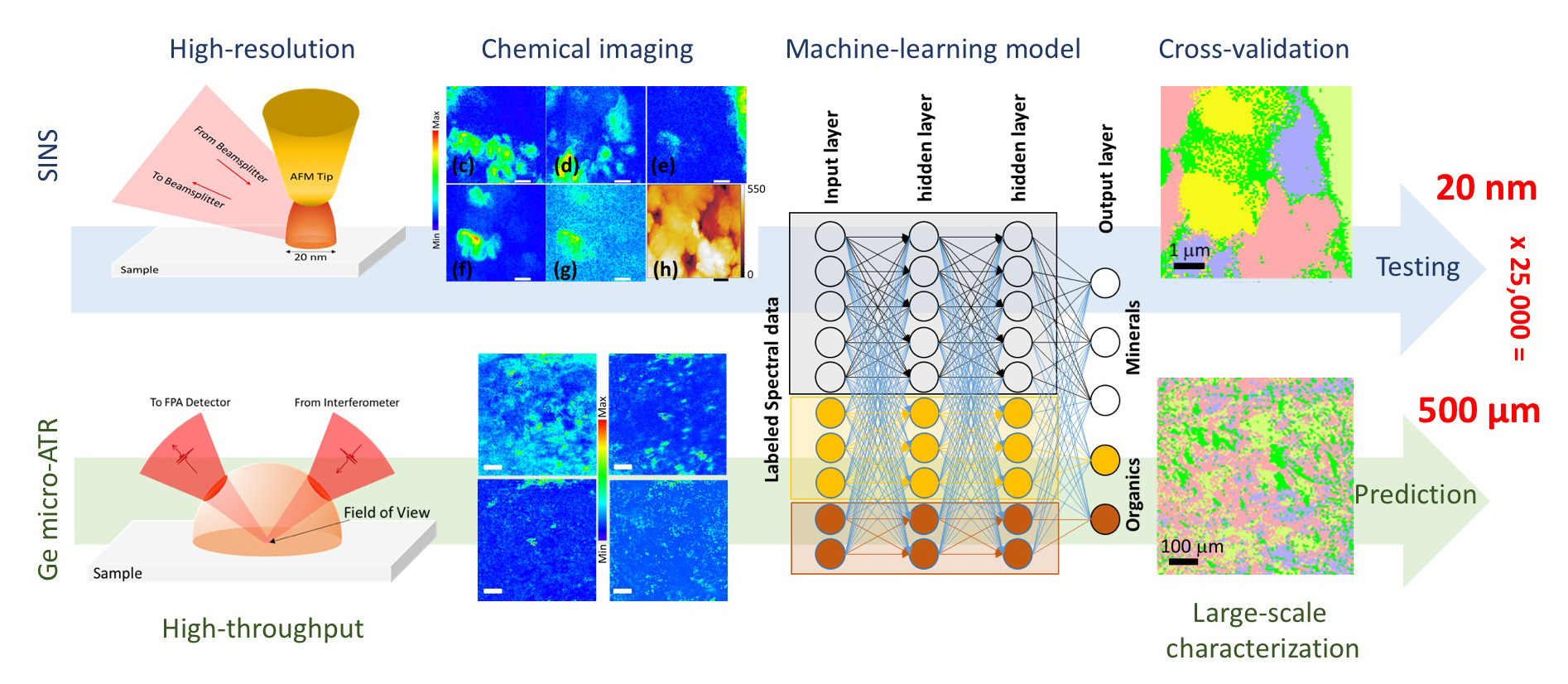
Z. Hao, H.A. Bechtel, T. Kneafsey, B. Gilbert, and P.S. Nico, “Cross-Scale Molecular Analysis of Chemical Heterogeneity in Shale Rocks,” Sci. Rep. 8, 2552 (2018), doi:10.1038/s41598-018-20365-6.
Adapted from the EESA highlight, “EESA Scientists Leverage Machine Learning to Connect Measurements of Shale Across Scales.”