Lithium-metal solid-state batteries are a promising technology, but the deposition (plating) of lithium metal on electrode surfaces remains a significant technical hurdle. In this work, researchers used micro-computed tomography (micro-CT) data to train an artificial intelligence model to identify characteristics vital to improving battery performance.
Researchers from Berkeley Lab’s Center for Advanced Mathematics for Energy Research Applications (CAMERA) led the development of “batteryNET,” a deep-learning algorithm that enables unprecedented assessment of lithium agglomeration in solid-state lithium-metal batteries. The interdisciplinary effort involved collaborators from the National Fuel Cell Research Center (NFCRC) at UC Irvine, UC Berkeley, the Advanced Photon Source (APS), and the Advanced Light Source (ALS). The model training was done at Berkeley Lab’s National Energy Research Scientific Computing Center (NERSC).
The training data consisted of micro-CT images, obtained at ALS Beamline 8.3.2 and the APS, of a lithium-metal battery as it charged and discharged. During this process, lithium ions become less mobile and start depositing at one of the electrode surfaces. As the deposits increase, dendritic structures can grow and penetrate the electrolyte that separates positive and negative electrodes, eventually short-circuiting the battery.
The batteryNET algorithm is able to categorize each pixel in the micro-CT images (about 5 gigabytes of data per frame) into classes or objects—a process called semantic segmentation. This allows researchers to turn high-resolution (but low-contrast) micro-CT data into information about the electrodes, electrolyte, and lithium distribution that can be used to inspect batteries for longer duration and safety.
“We have not only a snapshot but the whole evolution of the dendrites during a full cycle, so we can see that, at a certain amount of time and charge, problems start,” said Daniela Ushizima, a data scientist who leads CAMERA’s Computer Vision team. “Detecting and tracking dendrite formations can inform materials scientists about optimal electrode–electrolyte compositions and interactions.”
By combining operando x-ray computed tomography with the batteryNET algorithm, materials scientists gain an enhanced understanding of solid-state batteries’ inner workings. This semantic segmentation approach could enable more advanced optimization of battery designs, improving safety and allowing the exploration of the full potential of this energy storage technology.
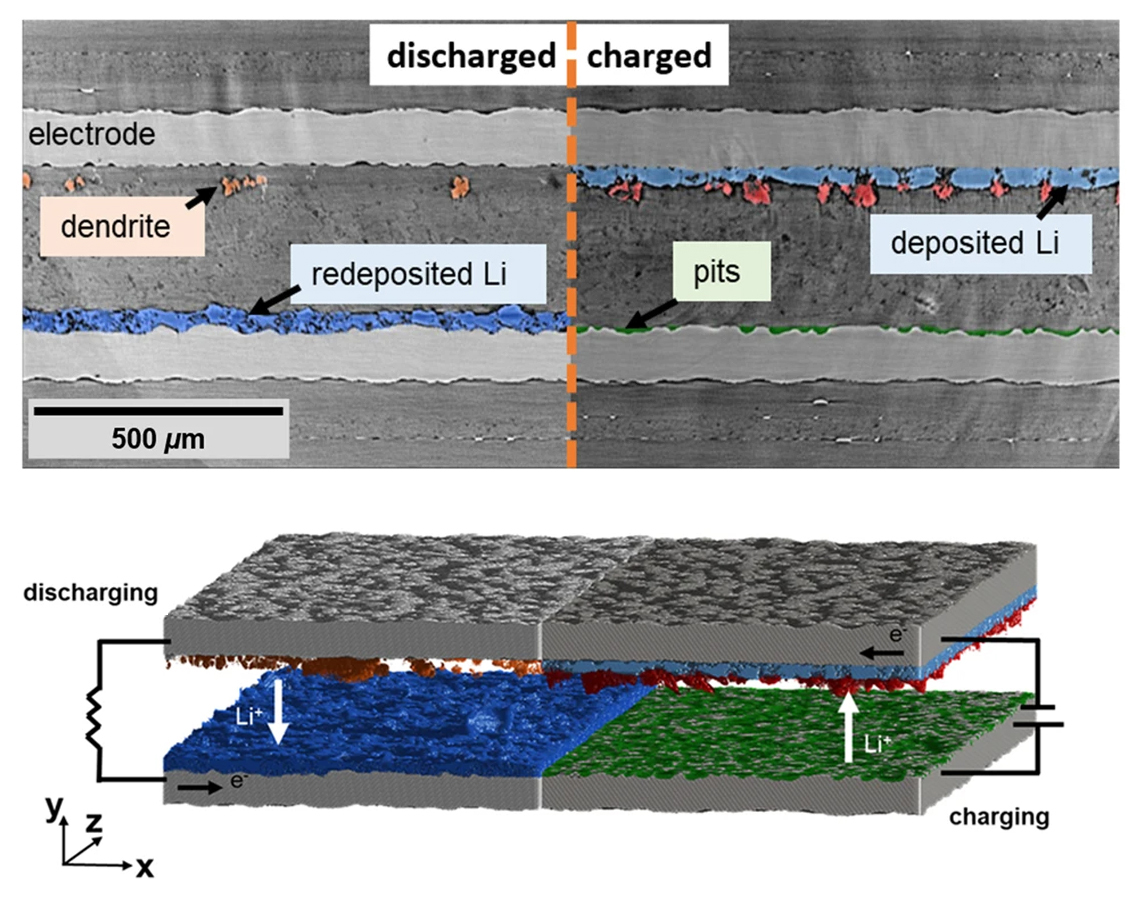
Y. Huang, D. Perlmutter, A.F.-H. Su, J. Quenum, P. Shevchenko, D.Y. Parkinson, I.V. Zenyuk, and D. Ushizima, “Detecting lithium plating dynamics in a solid-state battery with operando X-ray computed tomography using machine learning,” npj Comput. Mater. 9, 93 (2023); doi:10.1038/s41524-023-01039-y.
Adapted from the Berkeley Lab/Computing Research Division highlight, “Using Deep Learning to Better Assess Lithium Metal Battery Performance.”