SCIENTIFIC ACHIEVEMENT
Researchers used an artificial intelligence (AI) algorithm, similar to those used in natural-language (“chatbot”) models, to design a functional protein that was then structurally validated at the Advanced Light Source (ALS).
SIGNIFICANCE AND IMPACT
The work could speed the development of novel proteins for almost anything from therapeutics to degrading plastic.
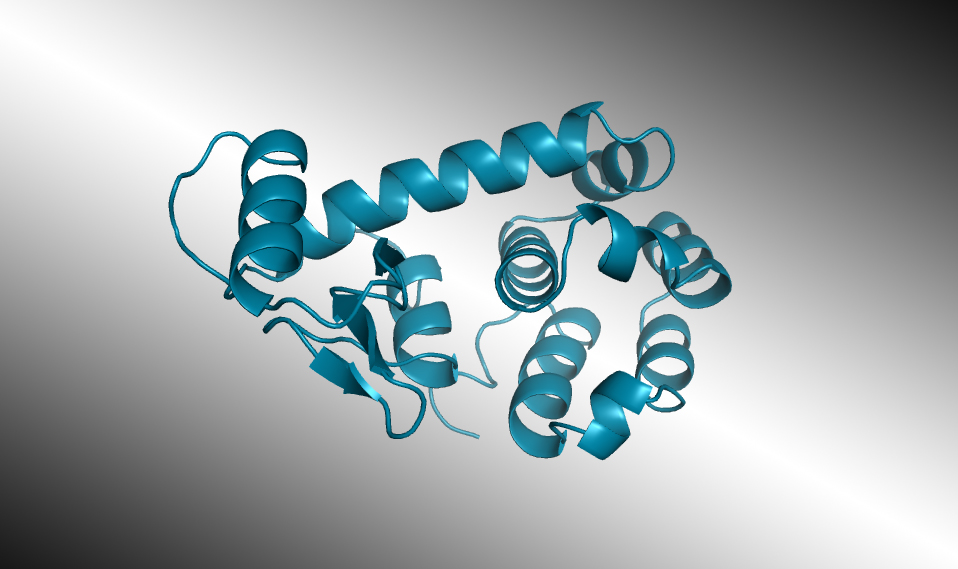
From sentences to sequences
Recently, efforts to create artificially generated text using so-called “chatbots” have grown increasingly sophisticated, rapidly progressing to the point where, given prompts for topic and style, a natural-language “AI” can produce credibly written output. Now, scientists have adapted this approach to generate artificial proteins—specifically, antibacterial enzymes called lysozymes.
Selected AI-designed lysozymes were synthesized and tested, and one structure was validated through protein crystallography at the ALS. In laboratory tests, some of the enzymes worked as well as those found in nature, even when their artificially generated amino-acid sequences diverged significantly from any known natural protein.
The experiment demonstrates the ability of natural-language models to capture at least some of the principles of biology. This new technology will energize the 50-year-old field of protein engineering by speeding the development of new proteins for almost anything from therapeutics to degrading plastic.
Learning the language of biology
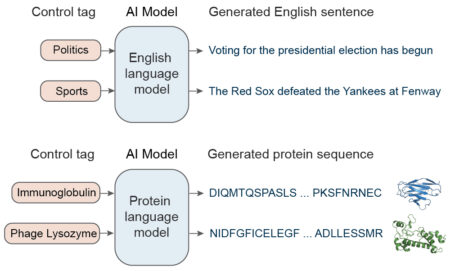
The AI used here, called ProGen, was developed by Salesforce Research to read and compose text. So-called “large language models” such as ProGen are trained on extremely large sets of writing samples. They pick the next word in a sentence based on a statistical analysis of word sequences in its training dataset. The larger the database, the better the results. Additionally, style tags associated with the desired output (e.g., politics or sports) can help the AI narrow the search for the best word in a given context.
A key insight of the Salesforce team was that proteins can be represented as a language made up of amino acids—the 20 molecules that make up every protein. They adapted ProGen to predict the probability of the next amino acid given the past amino acids in a raw sequence, with no explicit structural information or pairwise coevolutionary assumptions. To fine-tune its predictions, ProGen uses control tags covering things like protein family, molecular function, or biological process, which are available for a large fraction of sequences in public protein databases.
AI passes another test
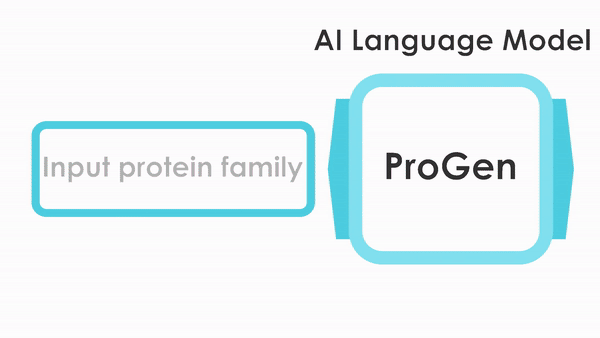
After being trained on a database of 280 million protein sequences, ProGen was asked to generate a completely new lysozyme. Of the million designs generated by the model, 100 promising candidate sequences were selected for real-world synthesis and study. Seventy-five of those displayed the desired antibacterial activity. Two were comparable in activity to a natural enzyme found in the whites of chicken eggs (known as hen egg white lysozyme, or HEWL). Similar lysozymes are found in human tears, saliva, and milk, where they defend against bacteria and fungi. Measured with x-ray crystallography at ALS Beamline 8.3.1, the atomic structure of one of the artificial enzymes looked just as predicted, even though its sequences were like nothing seen before.
In the near future, this process could be used to design highly tailored proteins with desired properties, such as the ability to bind to another molecule or the ability to operate at high temperatures, allowing the quick development of treatments for diseases or enzymes for industrial and environmental applications. More broadly, the work opens many new doors for utilizing state-of-the-art AI language modeling technology for accelerating protein engineering.
Contacts: Nikhil Naik and James Fraser
Researchers: A. Madani (Salesforce Research and Profluent Bio); B. Krause, C. Xiong, R. Socher, and N. Naik (Salesforce Research); E.R. Greene, J.L. Olmos Jr., and J.S. Fraser (UC San Francisco); S. Subramanian (UC Berkeley and Howard Hughes Medical Institute); B.P. Mohr and Z.Z. Sun (Tierra Biosciences); and J.M. Holton (Berkeley Lab, SLAC National Accelerator Laboratory, and UCSF).
Funding: Salesforce Research; University of California Office of the President; National Institutes of Health; Plexxikon Inc.; and U.S. Department of Energy (DOE), Office of Biological and Environmental Research, Integrated Diffraction Analysis Technologies program. Operation of the ALS is supported by DOE, Office of Science, Basic Energy Sciences program.
Publication: A. Madani, B. Krause, E.R. Greene, S. Subramanian, B.P. Mohr, J.M. Holton, J.L. Olmos Jr., C. Xiong, Z.Z. Sun, R. Socher, J.S. Fraser, and N. Naik, “Large language models generate functional protein sequences across diverse families,” Nat. Biotechnol. (2023), doi:10.1038/s41587-022-01618-2.
Adapted from the Berkeley Lab Biosciences Area highlight, “AI Technology Designs Novel Functional Enzyme,” the UCSF press release, “AI Technology Generates Original Proteins from Scratch,” and the Salesforce blog post, “Learning from evolution: Using AI language models to design functional artificial proteins.”
ALS SCIENCE HIGHLIGHT #480